P 12: T. Dandekar / E. Saliba
RNA-Omics and Single Cell-omics of human pathogens in 3D tissue ex vivo models
State of the art
The development of –omics technologies down to the single cell level has revolutionized our understanding of cellular identities and physiological states [1,4]. These technologies allowing to track the whole transcriptome, the nascent RNAs, the epigenome and the spatial positioning of the individual cells provide unique opportunities to understand the cellular tropism of pathogens and how a host and a pathogen control one and others [2,3]. The emergence of nascent RNAs sequencing at the single cell level [3] reveal differences between infected and uninfected cells with unpresented temporal resolution and could highlight the earliest transcriptomics changes after virus infection. Although we have used these technologies using monolayers of pathogens infecting homogeneous primary cells or cell lines [2,3], the development of engineered human tissues combined with single-cell –omics represent unique models to monitor the dynamics of human host-pathogen interactions at the closest of their reality. TD to add: Citation on systems biological modelling of OMICs data.
Previous work
The Saliba group pioneered the use of single cell technologies to track host-pathogen interactions. Notably we looked at the interaction of macrophages with Salmonella enterica [2] and revealed a unique mechanism of macrophage polarization manipulation by the bacteria (Fig.1). We demonstrated the possibility to perform single-cell RNA-seq on the eukaryotic pathogens Trypanosome brucei and on single-bacteria (not published). Recently we developed scSLAM-seq, a method to sequence nascent RNAs in the context of infection. In the context of the GRK, Saliba lab has already initiated many collaboration to decipher how pathogens interact with tissues among which the interaction of Salmonella with engineered intestinal tissue (Metzger lab) or the interaction of Trypanosome with skin tissue (Engstler). The Dandekar group models since a long time bacterial and other pathogen interactions with human cells during infection. We provide for each step bioinformatics expertise so that both regulatory and metabolic aspects of the pathogen in infection can be modelled as well as co-infection including different host cell types and responses (e.g. for Chlamydia primary polarized fallopian and cervical cells, cancer cells). Signalling, regulation and metabolism are modelled with high resolution. Boolean models capture decision processes in pathogen, innate immunity and host cells. Dynamical modelling includes semi-quantitative models and ODE-based detailed descriptions of cellular switches. Large-scale metabolic models will compare host and bacterial metabolism. Pathogen specific metabolism and regulation will be modelled building on our experience of analysing large-scale transcriptome datasets.
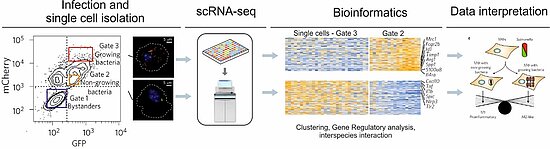
Figure1: : Illustration of single-cell sequencing workflow from an infection assay to the phenotypic analysis (based on [3]). Macrophages are infected with a fluorescence dilution plasmid carrying Salmonella strain that allows to differentiate macrophages with growing versus non-growing bacteria.
Work Plan
Hypotheses:
- Pathogens exhibit different tropism among different cell type and exhibit different behavior (replication, virulence) specific to each cell type
- Host-pathogen interaction of exclusive human pathogens relies at least in bacterial infections on typical pathways of pathogen invasion and typical pathways of human host cell defense
- We may even identify different types of defenses: epithelial barrier-type pathways, immune cell, particular dendritic cell type-specific defenses down to single cell level
- Bacterial secretion systems may mainly pave the way for invasion (e.g. in H.pylori)
- Alternatively, specific reprogramming of the host cell can be inferred using gene regulatory analysis (may be the case in Chlamydia)
By crossing different pathogen data, we expect to identify and classify infection patterns and design approaches to specifically boost immune defenses.
Experimental strategy. Across all the projects a tailored experimental single-cell -omics strategy will be established to specifically answer each question. Single-cell sorting will be handled using FACS at single-cell resolution. In order to perform single-cell RNA-seq, Smart-seqv4 and droplet-based sequencing (10x) will be used. In order to look at early time-point of infection, scSLAM-seq can be used. Other technologies, ATAC-seq to look at chromatin opening is currently in implementation in Saliba’s lab. Part of the GRK, Saliba’s lab will work in implementing spatial transcriptomics using slide-sequencing with high spatial resolution.
Data analysis strategy. Single-cell data will be analysed following workflows established in Dandekar’s and Saliba’s lab. Briefly, cell clustering will be performed using PCA, t-SNE and/or UMAP embedded in Seurat or RaceID. Gene network analysis can be performed using Scenic. scSLAM-seq will be analysed using GRAND-SLAM.
Dandekar provides analysis pipelines for these approaches [5-8]. All types of RNA sequencing data generated by Saliba will be analyzed after being preprocessed by the Saliba group. Our pipelines allow to characterize lnRNAs, miRNAs, and more detailed RNA studies in general, e.g. refined annotation, RNA secondary structure, RNA motif searches, and, most exciting, analysis of different types of RNA interactions (with proteins or RNA), differentiating between host and pathogen parts. Next, different proteins apparent from the data and expressed in the infection processes according to the data from experiment will be analyzed in detail (annotation, motifs, function, structure, domain composition). On these analyses we then build metabolic networks and will analyze available pathways for the pathogen and regarding specific host cells (in particular epithelical cells and immune cells such as dendritic cells) as well as regarding the dynamics of the infection process [6,7]. For the latter flux calculations will be based on proxy measures (time resolved RNAseq data or RT-PCR and protein expression data) or direct metabolite measurements. [6,8] Finally, regulatory interactions of the pathogen with the artificial human 3D tissue cells are modelled using software such as SQUAD and Jimena and dynamical models derived of the regulatory interactions during infection [5,7].
In this way we will describe host-pathogen interactions, protein interaction networks as well as the interactions with RNA and elucidate the systems biological implications for infection biology.
Proposed thesis topics:
Pathway modelling of host-pathogen interactions in pathogen A-Z of GRK
Systems biological modelling of pathogens in GRK (H. pylori, T. brucei, Salmonella
Metabolic modelling of pathogens in GRK (Chlamydia, Salmonella)
All this modelling will be based on the data generated by the cutting-edge Saliba group
Single Cell sequencing data reveal details of host-pathogen interactions on the above pathogens
Methods for single cell sequencing in infection biology studying pathogens of the GRK.
Planned co-operations in the GRK: Our platform, in particular single sequencing analysis but also related approaches such as dualRNAseq and other modifications and general RNAseq are available for all the consortium. In close collaboration with the experimental partners we will study host-pathogen interactions, starting from our own project on Chlamydia (coll. Prof. Rudel). In particular how different cells react to the infection as well as stage-specific information on the infection process of this intracellular pathogen will only be revealed by time-resolved single cell sequencing. In general (collaboration partners Rudel, Pavlovic, Sharma) bacterial infections and co-infections and their infection modes as well as cell-specific responses will be monitored (Trypanosomes: Engstler). For a number of pathogens including Chlamydia our technique will allow coinfection studies.
With the super-resolution microscopy (coll. with Prof. Sauer) we plan to have highest available resolution on the infection process by our complementary approaches using single-molecule FISH and expansion microscopy.
References
[1] Erhard F, …, Saliba AE, Dölken L. (2019) scSLAM-seq reveals core features of transcription dynamics in single cells. Nature. doi: 10.1038/s41586-019-1369-y.
[2 ]Müller LSM, …, Saliba AE, Sebra RP, Siegel TN. (2018) Genome organization and DNA accessibility control antigenic variation in trypanosomes. Nature. 563(7729):121-125.
[3] Saliba AE, …, Helaine S, Vogel J. (2016) Single-cell RNA-seq ties macrophage polarization to growth rate of intracellular Salmonella. Nat Microbiol. 2:16206.
[4] Saliba AE, …, Vogel J. (2014) Single-cell RNA-seq: advances and future challenges. Nucleic Acids Res. 42(14):8845-60.
[5] Breitenbach T, Liang C, Beyersdorf N, Dandekar T. Analyzing pharmacological intervention points: A method to calculate external stimuli to switch … steady states in regulatory networks. PLoS Comp. Biol. 2019 Jul16;15(7):e1007075.
[6] Srivastava M, Bencurova E, Gupta SK, Weiss E, Löffler J, Dandekar T. A. fumigatus challenged by Human Dendritic Cells: Metabolic and Regulatory Pathway Responses... Front Cell Infect Microb 2019 May 22;9:168.
[7] Dühring S, …Dandekar T, Schuster S. Modelling the host-pathogen interactions of macrophages and Candida albicans using Game Theory and dynamic optimization. J R Soc Interface. 2017 Jul;14(132). pii:20170095. doi: 10.1098/rsif.2017.0095.
[8] Winstel, V… Dandekar, T., Peschel, A., Xia G. (2013) Wall teichoic acid structure governs horizontal gene transfer between …bacterial pathogens. Nature Commun. 4,2345.