Research Pillar: AI in Digital Humanities
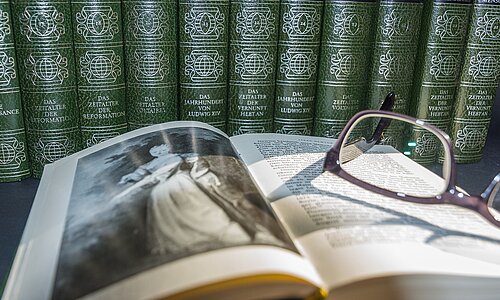
The research pillar "AI for Digital Humanities" aims to apply artificial intelligence techniques to the study of the humanities, specifically in the areas of musicology, geolingual studies, computational literary studies, multilingual natural language processing and digitization of cultural heritage.
The focus is on using cutting-edge AI methods, such as deep learning and representation learning, to gain new insights into the structures and patterns of human culture, as well as to democratize and make sustainable the use of state-of-the-art language technology for a diverse range of languages and cultures. The researchers in this pillar work on a wide range of topics, from extracting character networks from literary works, to analyzing language and space in megacities, to segmenting scans of old prints, to understanding the one-voice (monodic) music of the Middle Ages, to extracting data from tables in documents, to studying the works of a German Universal Scholar and more.
The goal is to bring together experts from different fields, such as computer science, linguistics, musicology, and history, to develop new methods and tools that can help scholars and researchers to analyze and understand various aspects of human culture and history.
Research Areas
Computational Literary Studies (CLS) have recently taken advantage of the latest developments in Natural Language Processing (NLP), with deep learning techniques bringing major improvements for tasks relevant to literature analysis: Examples include named entity recognition, anaphora and coreference resolution, sentiment analysis, scene detection or genre classification. At CAIDAS we leverage the latest advances in NLP to get insights into structures and patterns of literary works. Apart from NLP, machine learning has recently shown great potential in data that can be represented as graph or network. At CAIDAS weuse this techniques to study character networks, i.e. graphs where nodes represent literary characters and links. s. Combining these perspectives, in a recent work we study character networks extracted from a text corpus of J.R.R. Tolkien’s Legendarium. We show that this perspective helps us to analyse and visualise the narrative style that characterises Tolkien’s works. Addressing character classification, embedding and co-occurrence prediction, we further investigate the advantages of state-of-the-art Graph Neural Networks over a popular word embedding method. Our results highlight the large potential of graph learning in Computational Literary Studies.
Principal Investigators:
Publications:
- One Graph to Rule them All: Using NLP and Graph Neural Networks to analyse Tolkien's Legendarium.
2022. cite arxiv:2210.07871.
Vincenzo Perri, Lisi Qarkaxhija, Albin Zehe, Andreas Hotho and Ingo Scholtes.
[doi] [abstract] [BibTeX]
- Analysing Direct Speech in German Novels.
In: DHd 2018. 2018.
Fotis Jannidis, Leonard Konle, Albin Zehe, Andreas Hotho and Markus Krug.
[BibTeX]
- Annotation and beyond – Using ATHEN Annotation and Text Highlighting Environment.
In: DHd 2018. 2018.
Markus Krug, Ngoc Duyen Tanja Tu, Lukas Weimer, Isabella Reger, Leonard Konle, Fotis Jannidis and Frank Puppe.
[BibTeX]
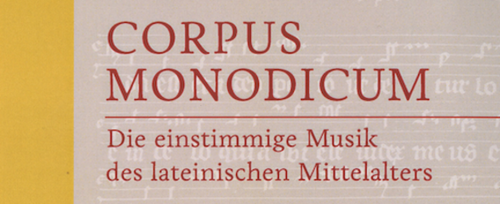
The research area "AI in Musicology" uses advanced computational techniques to analyze and understand various aspects of music. This can include the use of machine learning algorithms to analyze audio recordings, images, and video, with a focus on music information retrieval, audio signal processing, and machine learning. These techniques can be used to gain insights into the structure and patterns of music, for example, by identifying different sections of a song, or recognizing the style and genre of a piece of music. Additionally, the research area also includes the study of historical music, specifically the one-voice (monodic) religious and secular music with Latin text from the European Middle Ages. This research aims to understand the musical heritage of the Middle Ages, and the cultural context in which it was created, with the goal of providing new perspectives and insights into music through the application of AI and computational techniques.
Projects:
Principal Investigators:
Publications:
- Mid-level Harmonic Audio Features for Musical Style Classification.
In: Proceedings of the International Society for Music Information Retrieval Conference (ISMIR). Bengaluru, India, 2022.
Francisco C. F. Almeida, Gilberto Bernardes and Christof Weiß.
[BibTeX]
- Optical Medieval Music Recognition Using Background Knowledge.
Algorithms, 15(7):221, 2022.
Alexander Hartelt and Frank Puppe.
[doi] [BibTeX]
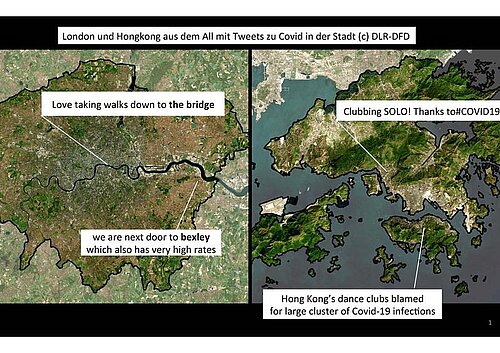
The "Geolingual Studies" research area focuses on understanding the relationship between language, cognition, emotion, and urbanization in megacities and smart cities. This includes examining how the urban environment affects human emotions, cognition and the way we use language. The research in this area uses methodologies from cognitive semantics, corpus-based analysis, multimodal discourse analysis and urban ecology, geoinformatics and digital humanities to generate evidence-based knowledge to contribute to making more livable cities. One part of the research focuses on the interconnections between human-nature relationships and the aesthetics of everyday urban places, while the other part focuses on the spatiotemporal characterization of the physical and social urban dimensions, aiming to unveil complex interconnections and counterintuitive relationships between humans and their main habitat: cities. The "Megacities" project is an example of this research area, led by researchers who are interested in comparing megacities such as London, New York and Mexico City to understand the similarities and differences between them.
Project:
Principal Investigator:
Multilingual Natural Language Processing (NLP) is a research field that develops computational methods and techniques for understanding and generating text in a multitude of human languages. This field combines techniques from computer science, linguistics, and machine learning to analyze, process and generate natural language text. CAIDAS researchers are specifically focused on developing sustainable, modular and sample-efficient NLP models that can be leveraged for low-resource languages (and the vast majority of the world's 7000+ languages are low-resource languages with very limited amounts of text data). They also work on interdisciplinary projects where they apply cutting-edge NLP methods to interesting problems from other disciplines, most prominently in the areas of Computational Social Science and Digital/Computational Humanities.
Principial Investigator:
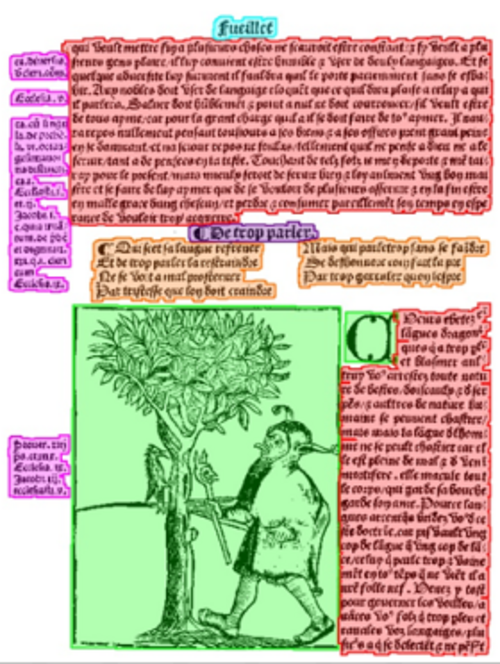
The "Digitization of Cultural Heritage" research area focuses on the use of technology to preserve and make accessible historical documents and artifacts. One of the key areas of focus within this field is the development of efficient and accurate methods for transcribing and digitizing printed texts from the past. This includes techniques for segmenting scans of old books and manuscripts into different regions, such as main text, marginalia, headings, and footers, as well as approaches for recognizing and transcribing text in the presence of challenging layouts. Additionally, the research also involves the development of optical document recognition (ODR) workflows for automating the transcription process and semantic annotation of the transcribed text. Another focus of this research is the study of historical figures and their works, with an emphasis on the development of efficient workflow for digitizing, transcribing and annotating early modern printed works, such as the works of Joachim Camerarius the Elder. The goal is to provide digital access to cultural heritage, by creating digital editions of historical texts, making them searchable, annotatable and linkable, and enabling the study of historical figures and their works in a new way.
Projects:
- Camerarius Digital
- Segmenation of Old Prints
- Corpus Monodicum
- Data Extraction From Tables in Documents
Principal Investigators:
Publications:
- KIETA: Key-insight extraction from scientific tables.
Applied Intelligence, 2022.
Sebastian Kempf, Markus Krug and Frank Puppe.
[doi] [BibTeX]
- Detecting Scenes in Fiction: A new Segmentation Task.
In: Proceedings of the 16th Conference of the European Chapter of the Association for Computational Linguistics: Volume 1, Long Papers. ACL, 2021.
Albin Zehe, Leonard Konle, Lea Dümpelmann, Evelyn Gius, Andreas Hotho, Fotis Jannidis, Lucas Kaufmann, Markus Krug, Frank Puppe, Nils Reiter, Annekea Schreiber and Nathalie Wiedmer.
[BibTeX]
Principial Investigators
Frank Puppe
Artificial Intelligence and Knowledge Systems
Ingo Scholtes
Machine Learning for
Complex Networks
Andreas Hotho
Data Science
Goran Glavaš
Natural Language
Processing
Carolin Biewer
English Linguistics
Hannes Taubenböck
Global Urbanization and
Remote Sensing
Fotis Jannidis
Computational Philology and Modern German Literary History
Christof Weiß
Computational Humanities